Projects Involved
Neural Mechanisms of Social Cognition and Decision-Making
1. Large-Scale Functional Connectivity Patterns Underlying Common Sense Perception (Behavior & fMRI study)
-
Investigated the neural mechanism underlying the process of judging whether a certain behavior or knowledge is common sense or not
-
Demonstrated that common sense judgment requires integrated neural involvements of a general memory retrieval network and social-affective regions, which include the amygdala, ventromedial prefrontal cortex, medial temporal lobe, angular gyrus, and hippocampus
[Find out More] *published (Ahn et al., 2018)*
2. Predicting the Effects of Task Difficulty Expectation on Subsequent Decision-Making (Behavior & fMRI study)
-
Investigated whether functional connectivity patterns during task preparation can predict the effects of task difficulty expectation on subsequent decision-making (giving up solving problems or not)
-
Demonstrated that participants tend to easily give up solving simple problems when a preceding cue indicates the subsequent problem will be “HARD” notwithstanding the cue is presented randomly
-
Classified participants who were largely affected by task difficulty expectation from those who were not using functional connectivity multivariate pattern analysis (fcMVPA) and support vector machine algorithm
-
Currently working on decoding motivation and predicting subsequent decisions (giving up or not) using activation patterns during task preparation periods
3. Voxel-wise Mapping of fMRI in Impression Anchoring and Adjustment (Behavior & fMRI study)
-
Performed voxel-wise mapping of fMRI data when impression anchoring or adjustment occurs
-
Functionally parcellated the orbitofrontal cortex, cingulate cortex, amygdala, and fusiform gyrus according to whether a voxel within the region is more involved with first impression anchoring or updating
Stress from Social Interaction and Alteration of Emotional Attention
1. Effects of Emotional Labor-Induced Chronic Stress on Emotional Attention (Behavior & fMRI study)
-
Recruited emotional laborers who had been suffered from chronic work-related stress
-
Demonstrated emotional laborers take longer to disengage from threatening facial stimuli
-
Demonstrated emotional attention and functional connectivity networks centering the amygdala, hippocampus, prefrontal cortex, and insula are altered in emotional labor group especially when perceiving threatening facial stimuli
[Poster]
Development of Novel Treatment Approaches for Clinical Groups
1. Development of a Smartphone Application to Reduce Social Anxiety
-
Configured Attentional Bias Modification (ABM) task as a mobile application
-
Currently validating the effects of the application on reducing anxiety level of social anxiety group
2. Development of Virtual Reality (VR) Training Program to Enhance the Cognitive Functions of the Elderly
-
Currently developing Activity of Daily Living (ADL) task to enhance the hippocampal functions of the elderly with
mild cognitive impairment (MCI)
3. Development of Non-Invasive Alternatives to the Wada Test (Epilepsy Patients Study)
-
Currently developing a fMRI memory paradigm and analysis pipeline as an alternative to the traditional Wada test during which one hemisphere of the brain should be anesthetized
Effects of Neurostimulation on Intrinsic Functional Networks and Cognition
1. Effects of Transcranial Direct Current Stimulation (tDCS) on Default Mode Network (tDCS & fMRI study)
-
Investigated whether tDCS with current intensity smaller than 0.5 mA and electrodes smaller than a conventional size
can induce alterations of default mode network (DMN)
-
Applied group independent component analysis (ICA)
-
Demonstrated frontopolar tDCS alters the intrinsic coactivation of regions within DMN
-
Currently exploring the effects and safety of tDCS on enhancing adolescents’ cognitive abilities, such as attentional allocation and memory
[Data Article] *published (Ahn et al., 2018)*
Optimizing Protocols for High-Resolution fMRI Data and Deep-Learning Approach
1. Multiband Echo Planar Imaging (EPI) for fMRI Data Acquisition
-
Applying multiband EPI (e.g., factors of 6) to obtain either 1) multiple slices of fMRI data simultaneously with the conventional acquisition time (i.e., TR = 2 sec) to create spatially high-resolution fMRI data (i.e., 1.5 mm isotropic voxel size), or 2) temporally sensitive fMRI data with shortened acquisition time (i.e., TR = 1 sec)
2. Reduction of Blood-Oxygen-Level-Dependent (BOLD) Signal Dropout in High-Resolution fMRI
-
Investigated the optimal high-resolution EPI protocols to reduce susceptibility-related BOLD signal dropout
-
Demonstrated that a high-resolution protocol with “slice tilt=30°, polarity of phase encoding direction = anterior to posterior (AP)” is optimal to reduce signal dropout except signals of the orbitofrontal cortex survive the most when a protocol with “slice tilt=parallel to anterior and posterior commissure line, polarity of phase encoding direction = AP” is applied.
3. Deep Learning Approach for Converting Low-Resolution fMRI Data into High-Resolution fMRI Data
-
Applying a deep-learning approach (convolutional neural network, CNN) to convert conventional low-resolution fMRI data (i.e., 3.75 × 3.75 × 4 mm voxel size) into high-resolution data (i.e., 1.5 mm isotropic voxel size) through super-resolution technique without modifying any fMRI acquisition protocols during an actual fMRI scanning period
[To Be Updated]
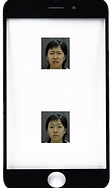
Resolution enhancement of T2*-weighted images using the CNN algorithm

