Neural Mechanisms of Social Cognition and Decision-Making
Impression anchoring and adjustment (Behavior & fMRI study)
-
Performed voxel-wise mapping of fMRI data when impression anchoring or adjustment occurs
-
Applied Least Squares Single (LSS) analysis and established two simple models representing impression anchoring or adjustment process using sign function
-
Functionally parcellated the orbitofrontal cortex, cingulate cortex, amygdala, and fusiform gyrus according to whether a voxel within the region is more involved with first impression anchoring or updating
**Master's Thesis ** (in preparation to submit to Social Cognitive and Affective Neuroscience)
Ahn, J., Nah, Y., & Han, S. Voxel-wise mapping of functional magnetic resonance imaging in impression formation
Committees:
1) Sanghoon Han, Ph.D. (advisor) Brain Cognition Lab of Memory and Decision-Making
2) Hackjin Kim, Ph.D. Laboratory of Social Decision Neuroscience
3) Young-Hoon Kim, Ph.D. Social & Cultural Psychology Lab
Abstract
In social dynamics, inconsistent information of others is often presented. It depends on person and situation which information one will focus on to impression of the target person. We conducted a functional magnetic resonance imaging (fMRI) study to comprehensively investigate the voxel-wise neural dynamics of anchoring and adjusting impression while incongruent information is provided. To aim that, we suggested a data-driven approach of model fitting analysis. Inside the MRI scanner, participants were shown male faces with four personality trait adjectives consecutively presented beneath. Two of adjectives described him positively whereas the other two described him negatively. After viewing all four adjectives, participants evaluated impression of the person on an eight-point scale. In fMRI analysis, each adjective presentation was considered as a single event and beta values of every event were estimated using general linear model. We then established two ideal models using simple sign functions. One of them hypothesized that beta estimates would decrease gradually during the presentation of the four adjectives (model 1) suggesting that a voxel was more involved with anchoring impression to the initial information. The other model hypothesized an increasing pattern of beta estimates (model 2) which assumed that neural activities would increase to monitor conflicts of information and adjust impression of the person based on incongruent information. Parameters that minimized a sum of squared deviations between ideal models and beta values were estimated using generalized reduced gradient (GRG) method. The results showed that voxel activities of the ventral anterior cingulate cortex (ACC), medial orbitofrontal cortex (OFC), posterior cingulate cortex, amygdala, and fusiform gyrus mainly fit model 1 better than model 2. We also identified that voxel activities of the dorsal ACC and lateral OFC fit into model 2 better than model 1. These findings demonstrate that the data-driven approach of model estimation successfully replicated previous impression studies. Also, the approach furthered our understanding of the neural dynamics of impression processing by functionally segmenting each region of interest according to their relative involvements in impression anchoring or adjustment.

Experimental Design
A face was paired with 4 personality trait adjectives consecutively presented beneath. Participants were asked to evaluate the impression of a man on an 8-point scale after viewing all 4 adjectives.
(a) Two positive adjectives were followed by 2 negative adjectives in half of the trials (PPNN condition). (b) The adjective presentation order was reversed in the other half of the trials (NNPP condition).
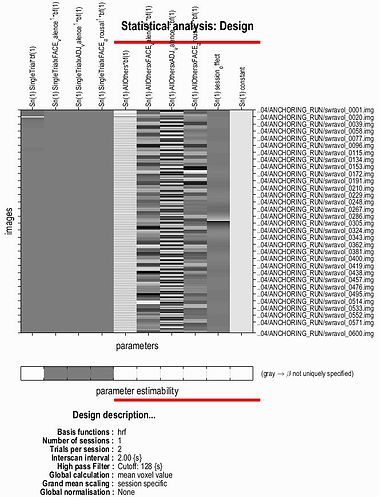
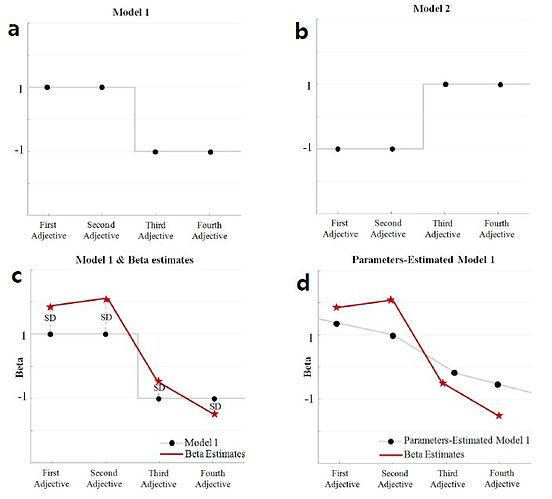
(Left) Design Matrix of Single-Trial Analysis: Beta-series estimation using Least Square Singles method (Mumford, Turner, Ashby, & Poldrack, 2012)
First, we considered every adjective presentation and impression evaluation as a single event of interest. Then, to calculate beta parameter estimates for every single event of interest and consider least of all the other single events, time course of the event of interest was modeled as the first regressor and those of all the other events were represented by additional regressor.
(Right) Model Fitting Analysis
(a) Four integers (1, 1, -1, -1) consisting model 1 are illustrated in black dots. The gray line is drawn only to indicate that 4 integers represent 4 consecutive events, and the line itself was not included in the analysis. Model 1 hypothesized decreasing voxel activity in response to incongruent information.
(b) Likewise, 4 discrete numbers (-1, -1, 1, 1) of model 2 hypothesized increasing voxel activity. Each digit of both models was respectively multiplied by parameters whose optimal value would be estimated in the next step.
(c) Four beta values of a voxel estimated in one trial are arbitrarily marked in red stars. Squared deviations between four betas and model 1 were calculated. This calculation was performed in every trial and all standard deviations (SD) were summed up in each condition. Nonlinear optimal parameters that minimized a sum of SD were estimated using GRG algorithm. Only model 1 is illustrated in this figure but the whole process was identically performed with model 2.
(d) Final parameters-multiplied models were estimated for every voxel separately in model 1 and 2. Only final model 1 is illustrated in this figure.
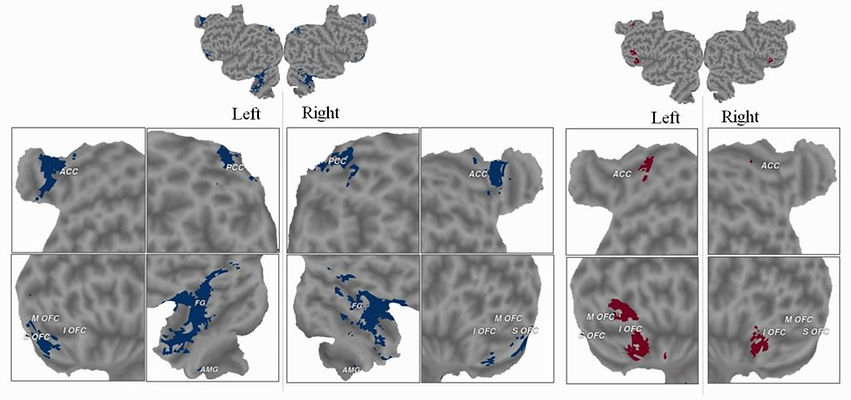
Parts of Main Results: Order-Sensitive Voxels
(a) Voxels of the bilateral superior and middle parts of the medial orbitofrontal cortex (OFC), ventral anterior cingulate cortex (ACC), posterior cingulate cortex (PCC), amygdala, and fusiform gyrus were more involved with processing first impression. They fit into model 1 better than model 2 in both PPNN and NNPP conditions. (b) Voxels of the lateral OFC and dorsal ACC significantly fit into model 2 regardless of conditions. They were more involved with detecting conflicts of impressions.
Reference
Mumford, J. A., Turner, B. O., Ashby, F. G., & Poldrack, R. A. (2012). Deconvolving BOLD activation in event-related designs for multivoxel pattern classification analyses. Neuroimage, 59(3), 2636-2643.